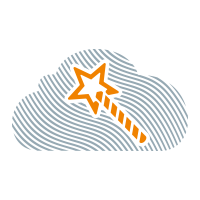
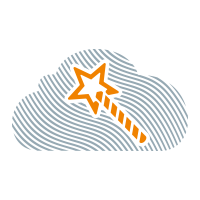
DBSec DBMasker-EnCloud
Product Overview
DBMasker as a masking product enables automatic identification and management of sensitive data, and provide flexible policy and masking scheme configurations to help enterprises quickly implement sensitive data masking processing, while ensuring the validity and availability of data, so that the data after masking can be safely applied to testing, development, analysis, and third-party usage environments.
DBMasker supports mainstream databases such as MySQL, Oracle, PostgreSQL, MSSQL, Informix, etc., supports multiple mainstream database application platforms such as Windows, Linux, AIX, and Solaris, and provides flexible masking rule configurations and masking rule extensions.
1) Addressing data sharing and exchange security
Through DBMasker's desensitization products, sensitive data exported from the production environment to development, testing, BI analysis and other environments has been desensitized, effectively preventing private data from flowing out of the enterprise without masking. Satisfying enterprises must not only protect private data, but also maintain regulatory compliance to meet corporate compliance.
2) Ensure the reliable operation of the business
DBMasker masking products provide the ability to follow the “guarantee that the data can be applied after masking” rule. This is reflected in the product's support in the following six areas:
Intelligence. With the growth of enterprise data and the continuous enrichment of data content, sensitive data may be scattered in hundreds or thousands of tables and fields, and the management of metadata is becoming more and more burdensome; at the same time, sensitive data extracted from business systems is highly arbitrary in terms of format and organization; therefore, masking products should have a good ability to identify sensitive data without relying on definitions of tables and fields in metadata, but automatically identify and effectively mask sensitive data based on data characteristics. For example, ID card, address, phone number, email, bank card number, license plate number, company name, etc.
Reversibility. With the popularity and popularization of data analysis, third-party analysts and internal subteams need to restore the analysis results based on desensitized data into business data to form real analysis results. Therefore, it is necessary to provide the ability to reverse restore data after masking.
Reproducible and unrepeatable, data masking requires providing the ability to repeatedly mask the same data. In different rounds of masking, ensure that the same private data is also the same after masking, so as to ensure that the data can be effectively correlated in an incremental environment. On the other hand, sometimes for safety reasons, it is necessary to provide an unrepeatable desensitization capability to ensure that the data generated by the same data in different rounds of masking is different, thus preventing reverse engineering from restoring the data.
Data validity. In order to ensure the normal operation of the business testing system and analysis system, it is necessary to ensure that the data after masking must accurately reflect the business attributes and data distribution characteristics of the original data; the information such as name, address, illness, and enterprise name in the original data needs to be readable even after masking; the desensitized data satisfies the data rules of the business system and can correctly pass the data validity verification of the business system, such as ID number, bank card number verification code, birthday data range, valid card issuer information, age and date of birth matching, etc.;
Data integrity, after masking, data needs to be guaranteed to be complete, and provide the ability to not change the size of the original data and not include invalid information to prevent sensitive data from not meeting the definition of target data, resulting in situations where it cannot be stored smoothly.
Data correlation, the data after masking should meet the data relationship characteristics of the business system, and the original data relationships are strictly preserved; for example, when ID numbers appear in multiple tables, it is necessary to ensure that these data are the same after masking. Also, for data with time series relationships, it is necessary to ensure that the original time series can be maintained even after each date is descaled.
Through support for the above six aspects, DBMasker effectively guarantees that the data after masking can meet the same business rules as the original data, is fictional data that represents actual business attributes, and enables users of the masking data to feel that the data is real from an experience point of view, thus ultimately ensuring that the use of desensitized data can guarantee the reliable operation of the business.
3) Automated processing improves desensitization efficiency
Through the automatic sensitive data discovery function, it is possible not only to avoid the extensive work caused by manually defining sensitive data, but also to ensure that no private information is missed, and new sensitive data fields can be continuously discovered.
The system has a variety of built-in masking algorithms. Appropriate masking algorithms are recommended for discovered sensitive data fields. Staff can make minor adjustments or use them directly.
The system supports scheduled tasks and can automatically execute masking tasks at a set time.
Through the above automated processing, it is possible to reduce a large amount of manual processing, reduce the error rate, and provide technical guarantees for safe desensitization.
This product supports the BYOL method and provides a seven-day free trial license. You can call the technical support hotline 400 923 1376 for help.
Version
By
安华金和 DBSECCategories
Operating System
Linux/Unix, CentOS release 6.10 (Final)
Delivery Methods